Integrasi Sistem Pengukuran Tanda-Tanda Vital Non Kontak Pada Security Robot
Sari
Robot sering kali digunakan untuk menggantikan tugas manusia saat membutuhkan ketelitian, kecepatan, jam kerja yang tinggi, dan kondisi yang berbahaya. Dalam pandemi COVID-19 peran robot dapat semakin penting. Salah satunya adalah robot security yang berfungsi melakukan pengamanan lingkungan. Robot security dapat difungsikan sebagai skrining awal kondisi kesehatan pengunjung suatu fasilitas umum dengan cara dilengkapi alat ukur tanda-tanda vital. Namun tanda-tanda vital tersebut harus dapat diukur secara non kontak. Sehingga dalam penelitian ini dibuat sebuah alat ukur tanda-tanda vital non kontak yang diletakan pada sebuah robot security. Tanda-tanda vital yang diukur meliputi suhu tubuh, detak jantung, dan frekuensi pernafasan. Dengan kata lain, penelitian ini melakukan integrasi pengukuran ketiga jenis tanda-tanda vital tersebut. Pengukuran suhu tubuh non kontak telah dibuat dengan cara menganalisis intensitas citra termal subjek di bagian wajah. Citra termal ini juga diproses untuk mendapatkan frekuensi pernafasan. Perubahan suhu di area lubang hidung merepresentasikan proses subjek saat menghirup dan menghembuskan udara pernafasan. Pengukuran detak jantung non kontak dilakukan dengan menggunakan teknik remote photoplenthysmograph. Algoritma yang digunakan adalah Plane Orthogonal to Skin (POS). POS bekerja dengan cara mendeteksi perubahan warna kulit wajah yang diakibatkan oleh aliran darah di bawah kulit wajah pada saat pemompaan darah oleh jantung. Berdasarkan hasil pengujian suhu tubuh, didapatkan tingkat error sebesar 0,4%. Tingkat error pengukuran frekuensi pernafasan adalah sebesar 4.72 %, sedangkan untuk detak jantung memiliki error sebesar 4,93%.
Robots are often used to replace humans in certain tasks. In general, when precision, speed, high working hours, and hazardous condition. Under conditions of the COVID-19, the role of robots can be even more important. One of them is a security robot that has functions to protect the environment. Security robot can be used as an initial screening for the health condition of visitors to a public facility by being equipped with vital sign measuring devices. However, these vital signs must be measured in a non-contact manner. In this study, non-contact vital signs measurement tool was created and placed on a security robot. Vital signs measured include body temperature, heart rate, and respiratory rate. In other words, this study integrates the measurements of the three types of vital signs. Non-contact body temperature measurements have been made by analyzing the thermal image of the subject. The subject's body temperature was measured based on the intensity thermal image on the face. This thermal image is also processed to obtain the respiratory frequency. Temperature changing in nostril area represent the subject's respiration process. Non-contact heart rate measurements were performed using remote photoplethysmograph. The algorithm used is Plane Orthogonal to Skin (POS). POS works by detecting changes of facial skin color that caused by blood flow under the skin when is pumped by heart. Based on body temperature testing, an error rate of 0.4% was obtained. The error rate for measuring the respiratory rate is 4,72%, while the heart rate has an error of 4,93%.
Kata Kunci
Teks Lengkap:
PDFDilihat:
Referensi
R. A. Firmansyah, Y. A. Prabowo, and T. Suheta, “Rancang Bangun Pengukur Detak Jantung Non Kontak Menggunakan Pencitraan Termal untuk Robot Security,” J. MEDIA Inform. BUDIDARMA, vol. 6, no. 1, Art. no. 1, Jan. 2022, doi: 10.30865/mib.v6i1.3416.
R. A. Firmansyah, Y. A. Prabowo, and T. Suheta, “Thermal Imaging-Based Body Temperature and Respiratory Frequency Measurement System for Security Robot,” Przegląd Elektrotechniczny, vol. 2022, no. 06, p. 126.
S. Muharom, S. Asnawi, and A. Bachri, “Robot Pengikut Target Berdasarkan Bentuk dan Warna Menggunakan Metode HSV Untuk Aplikasi Assistant Robot,” J. JE-UNISLA Electron. Control Telecomunication Comput. Inf. Power Syst., vol. 6, no. 1, Art. no. 1, Mar. 2021, doi: 10.30736/je.v6i1.571.
M. R. V. Aditya, N. L. Husni, D. A. Pratama, and A. S. Handayani, “Penerapan Sistem Pengolahan Citra Digital Pendeteksi Warna pada Starbot,” TEKNIKA, vol. 14, no. 2, Art. no. 2, Dec. 2020.
A. Ishida and K. Murakami, “Extraction of nostril regions using periodical thermal change for breath monitoring,” in 2018 International Workshop on Advanced Image Technology (IWAIT), Jan. 2018, pp. 1–5. doi: 10.1109/IWAIT.2018.8369727.
X. Li, Z. Zhang, and J. Wu, “Measurement of Respiration Rate Using Thermal Imaging,” in 2021 IEEE 5th Advanced Information Technology, Electronic and Automation Control Conference (IAEAC), Mar. 2021, pp. 2444–2449. doi: 10.1109/IAEAC50856.2021.9390916.
P. Jakkaew and T. Onoye, “Non-Contact Respiration Monitoring and Body Movements Detection for Sleep Using Thermal Imaging,” Sensors, vol. 20, no. 21, p. 6307, Nov. 2020, doi: 10.3390/s20216307.
L. Maurya, P. Mahapatra, and D. Chawla, “Simultaneous Breathing Monitoring of Multiple Persons Using Thermal and Visible Imaging,” IEEE Sens. J., vol. 21, no. 24, pp. 28057–28065, Dec. 2021, doi: 10.1109/JSEN.2021.3124615.
L. Chen, N. Liu, M. Hu, and G. Zhai, “RGB-thermal Imaging System Collaborated with Marker Tracking for Remote Breathing Rate Measurement,” in 2019 IEEE Visual Communications and Image Processing (VCIP), Dec. 2019, pp. 1–4. doi: 10.1109/VCIP47243.2019.8965987.
T. Negishi, G. Sun, H. Liu, S. Sato, T. Matsui, and T. Kirimoto, “Stable Contactless Sensing of Vital Signs Using RGB-Thermal Image Fusion System with Facial Tracking for Infection Screening,” in 2018 40th Annual International Conference of the IEEE Engineering in Medicine and Biology Society (EMBC), Jul. 2018, pp. 4371–4374. doi: 10.1109/EMBC.2018.8513300.
G. de Haan and V. Jeanne, “Robust Pulse Rate From Chrominance-Based rPPG,” IEEE Trans. Biomed. Eng., vol. 60, no. 10, pp. 2878–2886, Oct. 2013, doi: 10.1109/TBME.2013.2266196.
W. Wang, A. C. den Brinker, S. Stuijk, and G. de Haan, “Algorithmic Principles of Remote PPG,” IEEE Trans. Biomed. Eng., vol. 64, no. 7, pp. 1479–1491, Jul. 2017, doi: 10.1109/TBME.2016.2609282.
Q.-V. Tran, S.-F. Su, W. Sun, and M.-Q. Tran, “Adaptive Pulsatile Plane for Robust Noncontact Heart Rate Monitoring,” IEEE Trans. Syst. Man Cybern. Syst., vol. 51, no. 9, pp. 5587–5599, Sep. 2021, doi: 10.1109/TSMC.2019.2957159.
X. Liu, X. Yang, D. Wang, and A. Wong, “Detecting Pulse Rates From Facial Videos Recorded in Unstable Lighting Conditions: An Adaptive Spatiotemporal Homomorphic Filtering Algorithm,” IEEE Trans. Instrum. Meas., vol. 70, pp. 1–15, 2021, doi: 10.1109/TIM.2020.3021222.
R. Song, J. Li, M. Wang, J. Cheng, C. Li, and X. Chen, “Remote Photoplethysmography With an EEMD-MCCA Method Robust Against Spatially Uneven Illuminations,” IEEE Sens. J., vol. 21, no. 12, pp. 13484–13494, Jun. 2021, doi: 10.1109/JSEN.2021.3067770.
D. Cho, J. Kim, K. J. Lee, and S. Kim, “Reduction of Motion Artifacts From Remote Photoplethysmography Using Adaptive Noise Cancellation and Modified HSI Model,” IEEE Access, vol. 9, pp. 122655–122667, 2021, doi: 10.1109/ACCESS.2021.3106046.
B.-F. Wu, Y.-C. Wu, and Y.-W. Chou, “A Compensation Network With Error Mapping for Robust Remote Photoplethysmography in Noise-Heavy Conditions,” IEEE Trans. Instrum. Meas., vol. 71, pp. 1–11, 2022, doi: 10.1109/TIM.2022.3141149.
R. Song, S. Zhang, C. Li, Y. Zhang, J. Cheng, and X. Chen, “Heart Rate Estimation From Facial Videos Using a Spatiotemporal Representation With Convolutional Neural Networks,” IEEE Trans. Instrum. Meas., vol. 69, no. 10, pp. 7411–7421, Oct. 2020, doi: 10.1109/TIM.2020.2984168.
B.-F. Wu, P.-W. Huang, D.-H. He, C.-H. Lin, and K.-H. Chen, “Remote Photoplethysmography Enhancement with Machine Leaning Methods,” in 2019 IEEE International Conference on Systems, Man and Cybernetics (SMC), Oct. 2019, pp. 2466–2471. doi: 10.1109/SMC.2019.8914554.
H.-S. Hwang and E.-C. Lee, “Non-Contact Respiration Measurement Method Based on RGB Camera Using 1D Convolutional Neural Networks,” Sensors, vol. 21, no. 10, Art. no. 10, Jan. 2021, doi: 10.3390/s21103456.
P. Huang, C. Lin, M. Chung, T. Lin, and B. Wu, “Image based contactless blood pressure assessment using Pulse Transit Time,” in 2017 International Automatic Control Conference (CACS), Nov. 2017, pp. 1–6. doi: 10.1109/CACS.2017.8284275.
C. Barbosa Pereira, M. Czaplik, V. Blazek, S. Leonhardt, and D. Teichmann, “Monitoring of Cardiorespiratory Signals Using Thermal Imaging: A Pilot Study on Healthy Human Subjects,” Sensors, vol. 18, no. 5, May 2018, doi: 10.3390/s18051541.
T. Negishi et al., “Contactless Vital Signs Measurement System Using RGB-Thermal Image Sensors and Its Clinical Screening Test on Patients with Seasonal Influenza,” Sensors, vol. 20, no. 8, Art. no. 8, Jan. 2020, doi: 10.3390/s20082171.
DOI: https://doi.org/10.15575/telka.v10n2.109-121
Refbacks
- Saat ini tidak ada refbacks.
Jurnal TELKA terindex oleh :
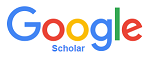
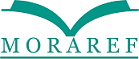
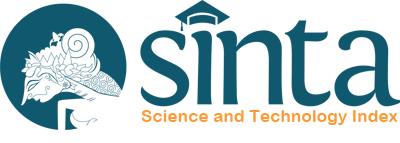
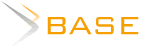
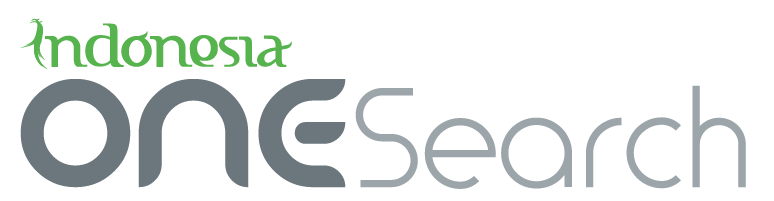
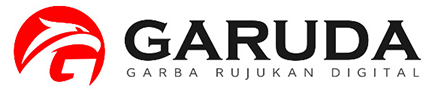
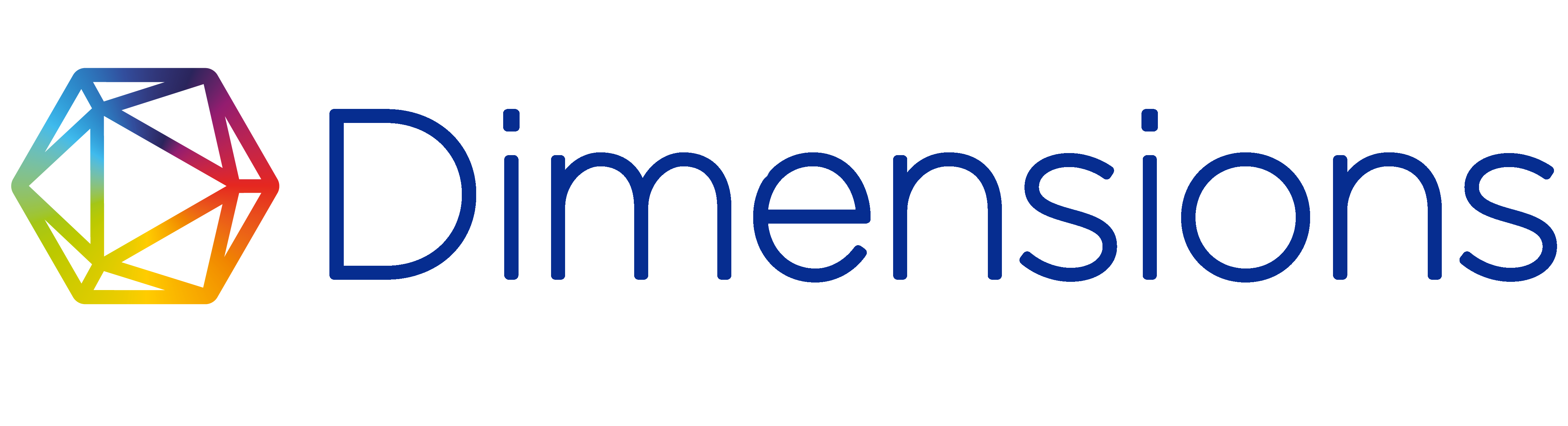
Didukung oleh :
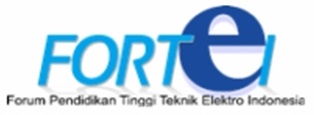

Ciptaan disebarluaskan di bawah Lisensi Creative Commons Atribusi-NonKomersial-BerbagiSerupa 4.0 Internasional.